- Frazis Capital Partners
- Posts
- September 2024 Investment Update
September 2024 Investment Update
The first rate cuts, a new scaling vector, and healthcare booms
Dear investors and well-wishers,
The fund returned 1.6% in August, taking us to 83% for the calendar year-to-date.
Rate cuts
Last week the US Federal Reserve cut their benchmark rate by 50 basis points. With markets close to highs, financial conditions are supportive.
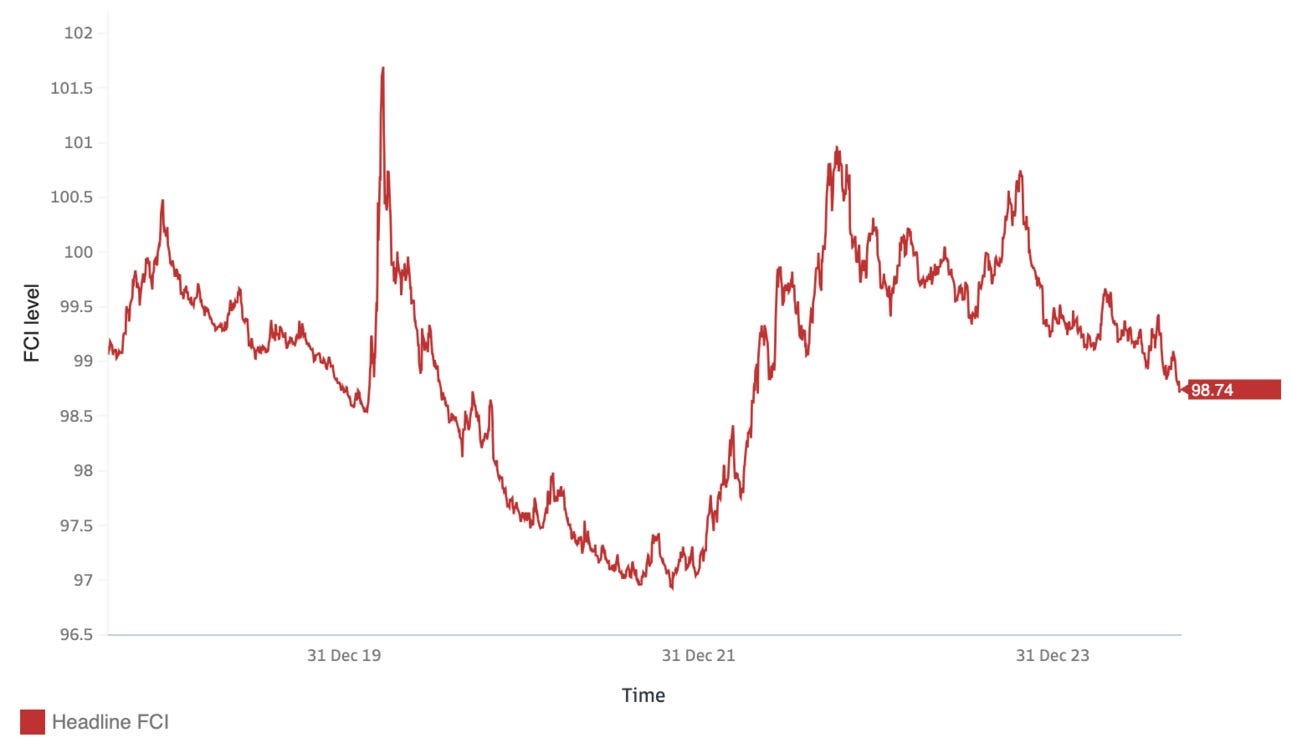
Goldman Sachs Financial Conditions Index, lower is easier/looser.
But real rates themselves are highly restrictive. Even after a 50 basis point cut, the US benchmark rate is 4.5%, way ahead of inflation at 2.5%.
Usually, the best scenario for stocks is a weak economy and a central bank trying to stimulate demand, as their blunt tools have an outsize effect on risk markets (in both directions).
The worst scenario is a wealth-destroying recession. There are certainly things to worry about - rising unemployment, falling GDP-per-capita, and consumer weakness for a start - we are not there yet. And the Fed’s restrictive stance leaves plenty of room for stimulus.
The action is still in semiconductors
OpenAI’s latest model update is an important one, incorporating planning steps directly into the model.
It’s likely no coincidence that Noam Brown from OpenAI released a talk on the ‘Parables of Planning’ only five days ago.
He studies the application of state-of-the-art AI to games.
Games played an important role in the history of AI. Victories in Chess and Go marked major advances in capabilities. And now, by integrating planning, computers are beating humans in new domains.
Without giving too much away from this excellent talk, I wouldn’t play online poker right now…
Towards the end Noam discusses the trade-off between applying compute to training, and applying it at run-time inference.
The optimal strategy for companies building mass-market LLMs is to invest as much compute into training as possible, then deliver it as cheaply as possible to hundreds of millions of users.
But many problems are better attacked by allocating more compute at run-time, and incorporating multiple steps of planning.
This is exactly what OpenAI’s new models offer. They spend far more time ‘thinking’*, resulting in better answers in areas where LLMs have struggles, notably mathematics (more training and artificial data sets have also probably helped here).
Responses to some of my own queries have taken over 75 seconds, which is long when you consider Google can index the entire internet and serve it on your screen in milliseconds.
So we now have a second scaling vector: increasing the compute available at inference for hundreds of millions of people.
In other words, another step-change in demand for compute.
And that’s not to say the benefits of training are plateauing. Applying more compute to training is still leading to incremental advances, which is why there is talk of trillion dollar clusters, and Microsoft is restarting nuclear reactors to supply the necessary power.
AI in software
Klarna announced it’s replacing Workday and Salesforce with custom software. You may recall Klarna made headlines early on in the GPT era by announcing two thirds of their customer service calls were being handled by ChatGPT, in place of 700 employees.
The software industry (and its investors) now have to face this new threat.
It makes sense - platforms like Salesforce requires pretty serious customization, so why not just build that functionality in-house?
This is a bitter pill for software investors. In the boom, profits were captured almost entirely by management and staff, with investors banking on future growth, customer stickiness, and immense cash flows in the near term.
With a few very notable exceptions, this did not come. Now every buyer is laser-focused on reducing cloud spend, and former darlings like Datadog and Snowflake are facing cut-throat competition from open-source alternatives.
And thanks to AI, the cost to run open-source alternatives or build solutions from scratch is cheaper than ever.
Software companies are responding by heralding their own efforts in AI. Salesforce, for example, is experimenting with autonomous agents.
But it remains to be seen if this improves economics or just drags on profits, and agents ultimately become as commoditized as large language models themselves.
And I suspect it’s easier to spin up your own AI salesbot than torture one from Salesforce into doing what you want.
Nearly three years after the 2021 peak, software indices are still down over 50%.
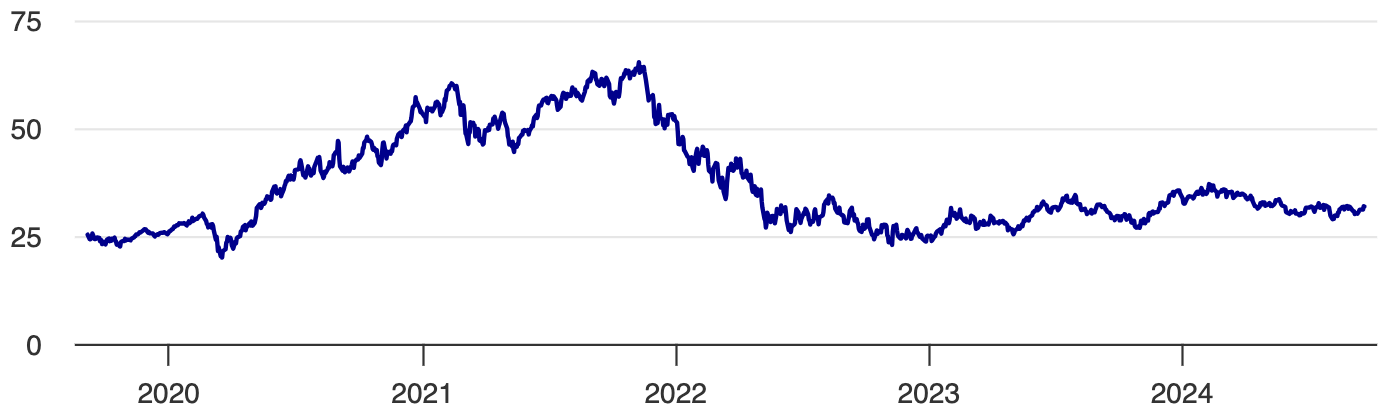
WCLD, a cloud computing ETF, is still down over 50% since 2021
At some point this will turn, but only with GAAP profit growth and multipels that can excite generalist investors. Sofware specialists will likely see a net capital outflow. As a cautionary tale Chinese tech is now negative over almost every time period since 2014.
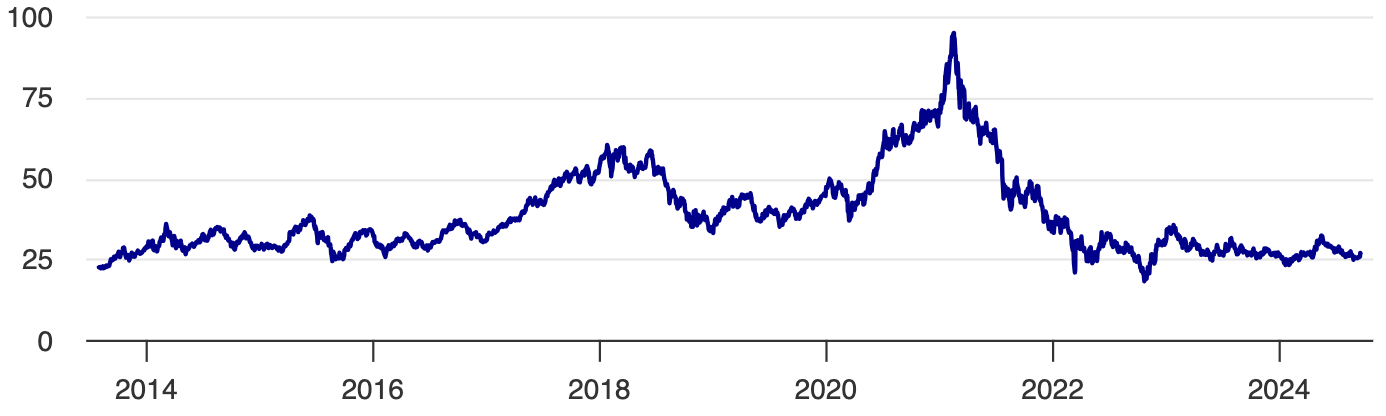
KWEB, Chinese internet ETF holding Alibaba, Tencent, Meituan, JD.com, etc
Portfolio
We were lucky to have positive returns in July and August, as a number of our largest positions dropped substantially. Our risk model gave us timely exits on both ELF and Celsius. We now have a large bank of situations where our AI-generated risk framework has markedly improved our returns.

A timely exit from Celsius (red triangle), resulting in a small profit instead of a large loss.
Healthcare was again a highlight. Our two largest positions, Clarity and Transmedics, are at or close to their highs. RxSight performed strongly through the pullback. And we are focusing more and more on ASX biotech opportunities, particularly those that do their own science in-house and have developed their own assets.
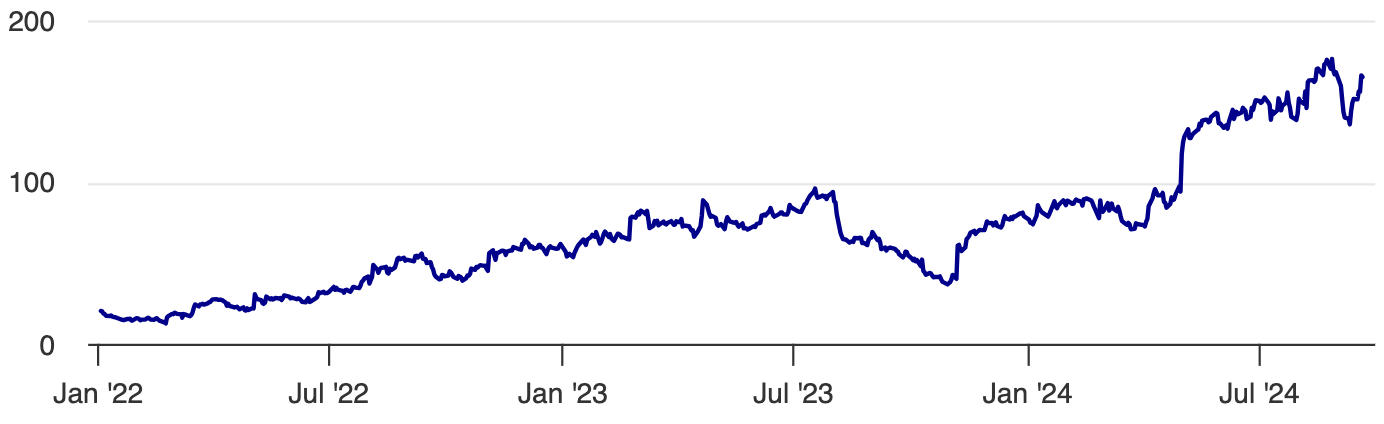
Transmedics
45% of our fund is now in healthcare, and more than half the rest is allocated to semiconductors.
Best regards
Michael
*This is similar to improving LLM results by asking the model to first structure a prompt, then sketch the outline of a solution, fill in the gaps, and finally review its own results. The latest models also incorporate new training data and are much better at coding and mathematics. Mathematics is an area where ‘synthetic data’ is most effective, as you can easily generate accurate question and answer pairs to train.